Research Interests
Near-Field Wireless Communications
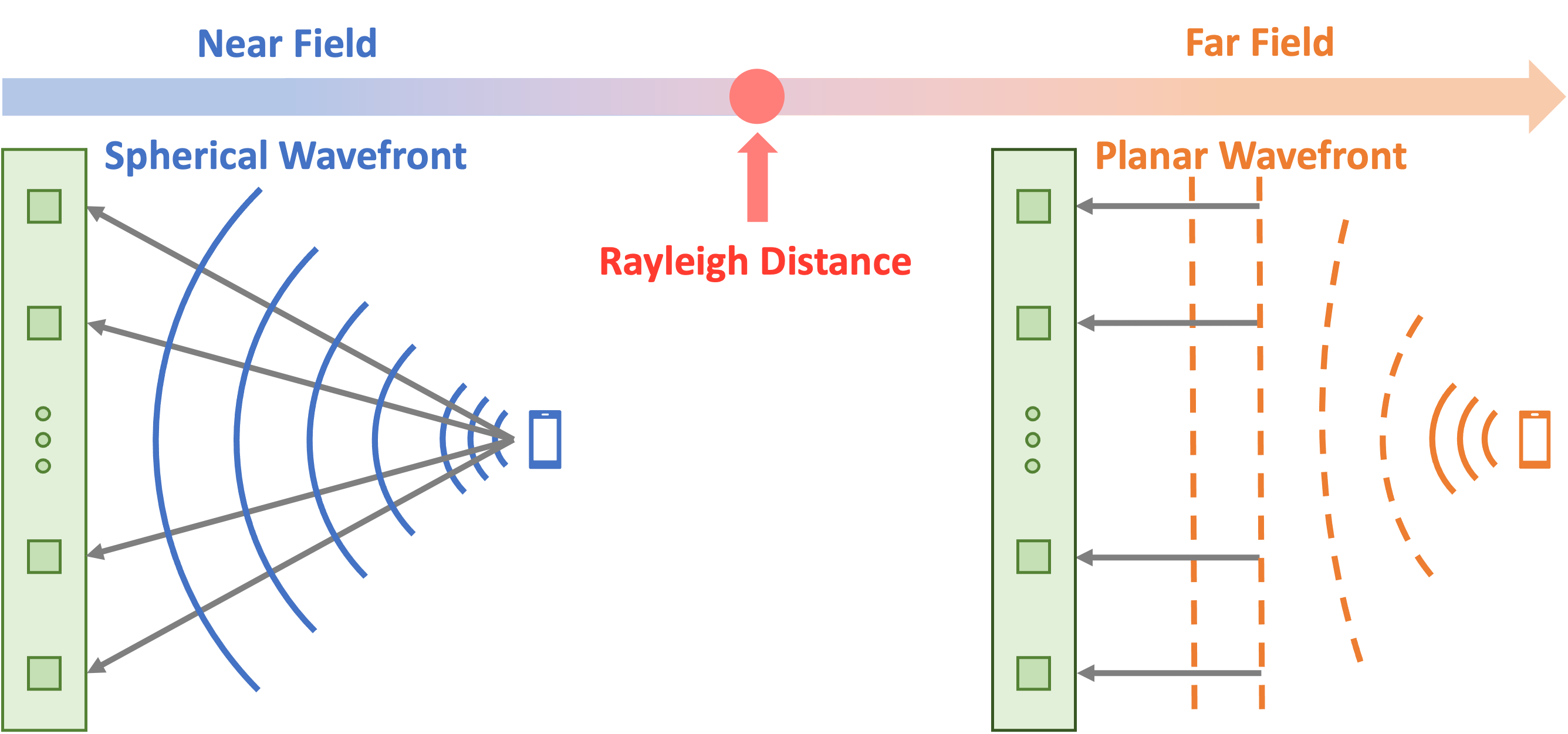
Leveraging extremely large MIMO (XL-MIMO) at mm-wave/THz bands in future 6G systems is not merely a quantitative increase in both antenna size and carrier frequency, but more importantly, a qualitative paradigm shift from conventional far-field communications to its near-field counterpart. In particular, the enlarged antenna aperture and shortened wavelength jointly expand the region defined as the near field, which was normally overlooked in
traditional wireless communications.
Y. Chen, Z. Ren, X. Yu, L. Liu, and J. Xu, “Exploiting moving arrays for near-field sensing,” IEEE Wireless Commun. Lett., vol. 14, no. 3, pp. 601-605, Mar. 2025. [Paper]
S. Liu, X. Yu, Z. Gao, J. Xu, D. W. K. Ng, and S. Cui, “Sensing-enhanced channel estimation for near-field XL-MIMO systems,” IEEE J. Sel. Areas Commun., Special Issue on Next Generation Advanced Transceiver Technologies, vol. 43, no. 3, pp. 628-643, Mar. 2025. [Paper] [Codes]
S. Liu and X. Yu, “Low-complexity near-field localization with XL-MIMO sectored uniform circular arrays,” accepted to IEEE Global Commun. Conf. (GLOBECOM), Cape Town, South Africa, Dec. 2024, pp. 4756-4761.
S. Liu, X. Yu, Z. Gao, and D. W. K. Ng, “DPSS-based codebook design for near-field XL-MIMO channel estimation,” in Proc. IEEE Int. Conf. Commun. (ICC), Denver, CO, USA, June 2024, pp. 3864-3870.
W. Yu, H. He, X. Yu, S.H. Song, J. Zhang, R. Murch, and K. B. Letaief, “Bayes-optimal unsupervised learning for channel estimation in near-field holographic MIMO,” IEEE J. Sel. Topics Signal Process., Special Issue on Near-Field Signal Processing: Algorithms, Implementations and Applications, vol. 18, no. 4, pp. 714-729, May 2024. [Paper]
R. Cao, W. Yu, H. He, X. Yu, S.H. Song, J. Zhang, Y. Gong, and K. B. Letaief, “Newtonized near-field channel estimation for ultra-massive MIMO systems,” in Proc. IEEE Wireless Commun. Netw. Conf. (WCNC), Dubai, UAE, Apr. 2024, pp. 1-6.
W. Yu, Y. Shen, H. He, X. Yu, J. Zhang, and K. B. Letaief, “Hybrid far- and near-field channel estimation for THz ultra-massive MIMO via fixed point networks,” in Proc. IEEE Global Commun. Conf. (GLOBECOM), Rio de Janeiro, Brazil, Dec. 2022, pp. 5384-5389.
R. Cao, H. He, X. Yu, J. Zhang, S.H. Song, Y. Gong, and K. B. Letaief, “Belief propagation for near-field cooperative localization and tracking in 6G vehicular networks,” in Proc. IEEE Int. Mediterranean Conf. Commun. Netw. (MeditCom), Athens, Greece, Sept. 2022, pp. 160-165.
Integrated Sensing and Communications (ISAC)
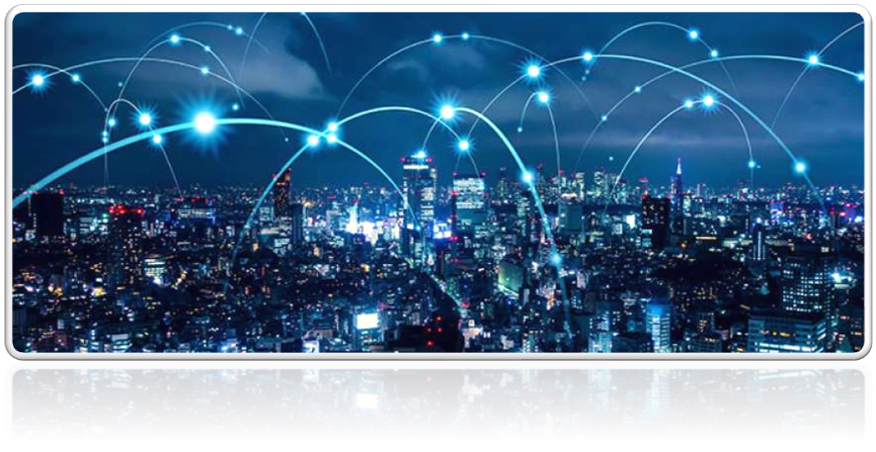
In 6G systems, not only high-quality wireless connectivity but also an accurate and powerful sensing capability, e.g., localization, mapping, and tracking, is required. Equipped with the sensing functionality as a native capability, future 6G wireless networks are anticipated to open their “eyes” and become perceptive networks. Therefore, the integration of sensing and communication (ISAC) will be one of the core components of 6G that will differentiate it from 5G. This also heralds a revolutionary paradigm shift from the communication-centric 5G wireless systems towards multi-functional 6G perceptive wireless networks.
X. Song, X. Qin, X. Yu, J. Xu, and D. W. K. Ng, “Active-IRS-enabled target detection,” submitted to IEEE Wireless Commun. Lett., Feb. 2025. [Paper]
X. Ye, Y. Mao, X. Yu, S. Sun, L. Fu, and J. Xu, “Integrated sensing and communications for low-altitude economy: A deep reinforcement learning approach,” submitted to IEEE Trans. Wireless Commun., Dec. 2024. [Paper]
X. Song, Y. Fang, F. Wang, Z. Ren, X. Yu, Y. Zhang, F. Liu, J. Xu, D. W. K. Ng, R. Zhang, and S. Cui, “An overview on IRS-enabled sensing and communications for 6G: Architectures, fundamental limits, and joint beamforming designs,” accepted to Sci. China Inf. Sci., Mar. 2025. [Paper]
X. Ye, Y. Mao, X. Yu, and L. Fu, “Intelligent omni-surface-aided integrated sensing and communications based on deep reinforcement learning with knowledge transfer,” IEEE Trans. Wireless Commun., to appear. [Paper]
Z. Hao, Y. Fang, X. Yu, J. Xu, L. Qiu, L. Xu, and S. Cui, “Energy-efficient hybrid beamforming with dynamic on-off control for integrated sensing, communications, and powering,” IEEE Trans. Commun., vol. 73, no. 3, pp. 1709-1725, Mar. 2025. [Paper]
Y. Fang, X. Yu, and J. Xu, “Joint transmit and reflective beamforming for multi-active-IRS-assisted cooperative sensing,” in Proc. Int. Conf. Wireless Commun. Signal Process. (WCSP), Hefei, China, Oct. 2024, pp. 181-186.
D. Xu, Y. Xu, X. Zhang, X. Yu, S.H. Song, and R. Schober, “Interference mitigation for network-Level ISAC: An optimization perspective,” IEEE Commun. Mag., vol. 62, no. 9, pp. 28-34, Sept. 2024. [Paper]
Y. Fang, S. Zhang, X. Li, X. Yu, J. Xu, and S. Cui, “Multi-IRS-enabled integrated sensing and communications,” IEEE Trans. Commun., vol. 72, no. 9, pp. 5853-5867, Sept. 2024. [Paper]
Y. Fang, X. Yu, J. Xu, and Y.-J. A. Zhang, “Multi-active-IRS-assisted cooperative sensing: Cramér-Rao bound and joint beamforming design,” submitted to IEEE Trans. Wireless Commun., June 2024. [Paper]
D. Xu, A. Khalili, X. Yu, D. W. K. Ng, and R. Schober, “Integrated sensing and communication in distributed antenna networks,” in Proc. IEEE Int. Conf. Commun. Wkshps. (ICC Wkshps), Rome, Italy, May 2023, pp. 1457-1462.
D. Xu, X. Yu, D. W. K. Ng, A. Schmeink, and R. Schober, “Robust and secure resource allocation for ISAC systems: A novel optimization framework for variable-length snapshots,” IEEE Trans. Commun., vol. 70, no. 12, pp. 8196-8214, Dec. 2022. [Paper]
L. Xie, X. Yu, and S.H. Song, “Intelligent reflecting surface-assisted maneuvering target sensing: True velocity estimation,” in Proc. Int. Symp. Wireless Commun. Syst. (ISWCS), Hangzhou, China, Oct. 2022. (Invited Paper) [Paper]
Interplay between Deep Learning and Communications
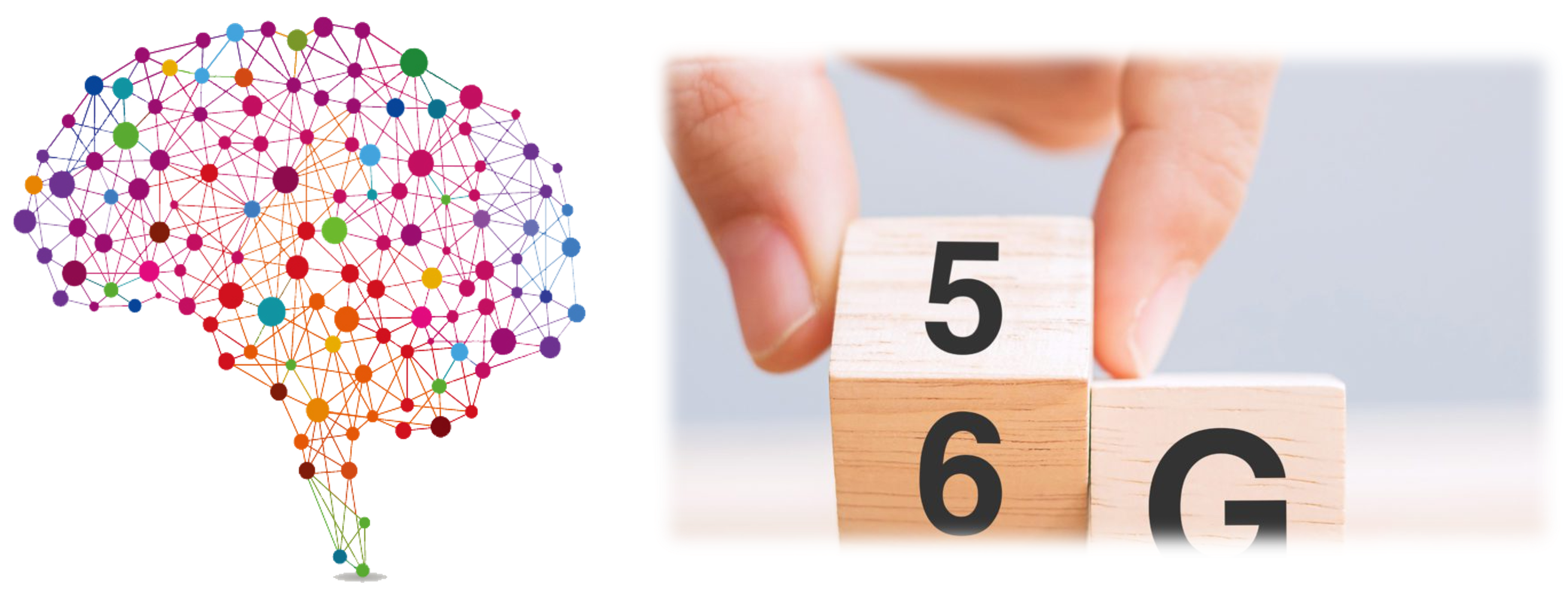
As future wireless networks are expected to experience an unprecedented level of complexity, we are rapidly reaching the point that exceeds the capabilities of traditional modeling and design approaches. Inspired by the recent success of deep learning in a variety of domains, deep learning-based algorithms have been proposed to design wireless systems, thriving in the emerging research area of “learning to communicate”. On the other hand, learning over networks opens up a new machine learning approach, federated learning, which collaboratively learns a shared prediction model while keeping all the training data on devices.
X. Ye, Y. Mao, X. Yu, and L. Fu, “Joint MCS adaptation and beamforming design for multi-user MISO systems: A constrained hybrid deep reinforcement learning approach,” submitted to IEEE Trans. Commun., Mar. 2025.
X. Ye, X. Song, X. Yu, and L. Fu, “Unlicensed millimeter-Wave NR-U and WiGig coexistence: A hybrid deep reinforcement learning approach,” submitted to IEEE Trans. Commun., Mar. 2025.
X. Ye, X. Yu, and L. Fu, “Digital twin enhanced deep reinforcement learning for intelligent omni-surface configurations in MU-MIMO systems,” IEEE Internet Things J., vol. 12, no. 9, pp. 13005-13020, May 2025. [Paper]
Y. Mao, X. Yu, K. Huang, Y.-J. A. Zhang, and J. Zhang, “Green edge AI: A contemporary survey,” Proc. IEEE, vol. 112, no. 7, pp. 880-911, July 2024. [Paper]
W. Yu, H. He, X. Yu, S.H. Song, J. Zhang, R. Murch, and K. B. Letaief, “Learning Bayes-optimal channel estimation for holographic MIMO in unknown EM environments,” in Proc. IEEE Int. Conf. Commun. (ICC), Denver, CO, USA, June 2024.
Y. Ma, W. Yu, X. Yu, J. Zhang, S.H. Song, and K. B. Letaief, “Lightweight and flexible deep equilibrium learning for CSI feedback in FDD massive MIMO,” in Proc. IEEE Int. Conf. Mach. Learn. Commun. Netw. (ICMLCN), Stockholm, Sweden, May 2024, pp. 299-304.
H. He, X. Yu, J. Zhang, S.H. Song, and K. B. Letaief, “Message passing meets graph neural networks: A new paradigm for massive MIMO systems,” IEEE Trans. Wireless Commun., vol. 23, no. 5, pp. 4709-4723, May 2024. [Paper]
W. Yu, Y. Shen, H. He, X. Yu, S.H. Song, J. Zhang, K. B. Letaief, “An adaptive and robust deep earning framework for THz ultra-Massive MIMO channel estimation,” IEEE J. Sel. Topics Signal Process., Special Issue on Advanced Signal Processing for Terahertz Communications in 6G and Beyond Networks, vol. 17, no. 4, pp. 761-776, July 2023. [Paper]
W. Yu, H. He, X. Yu, S.H. Song, J. Zhang, and K. B. Letaief, “Blind performance prediction for deep learning based ultra-massive MIMO channel estimation,” in Proc. IEEE Int. Conf. Commun. (ICC), Rome, Italy, May 2023, pp. 2613-2618.
H. He, A. Kosasih, X. Yu, J. Zhang, S.H. Song, W. Hardjawana, and K. B. Letaief, “GNN-enhanced approximate message passing for massive/ultra-massive MIMO detection,” in Proc. IEEE Wireless Commun. Netw. Conf. (WCNC), Glasgow, Scotland, UK, Mar. 2023, pp. 1-6.
Y. Ma, X. Yu, J. Zhang, S.H. Song, and K. B. Letaief, “Augmented deep unfolding for downlink beamforming in multi-cell massive MIMO with limited feedback,” in Proc. IEEE Global Commun. Conf. (GLOBECOM), Rio de Janeiro, Brazil, Dec. 2022, pp. 1721-1726.
Z. Qiao, Y. Shen, X. Yu, J. Zhang, S.H. Song, and K. B. Letaief, “Content-aware client selection for federated learning in wireless networks,” in Proc. IEEE Int. Mediterranean Conf. Commun. Netw. (MeditCom), Athens, Greece, Sept. 2022, pp. 49-54.
Y. Ma, Y. Shen, X. Yu, J. Zhang, S.H. Song, and K. B. Letaief, “Learn to communicate with neural calibration: Scalability and generalization,” IEEE Trans. Wireless Commun., vol. 21, no. 11, pp. 9947-9961, Nov. 2022. [Paper]
Y. Ma, Y. Shen, X. Yu, J. Zhang, S.H. Song, and K. B. Letaief, “Neural calibration for scalable beamforming in FDD massive MIMO with implicit channel estimation,” in Proc. IEEE Global Commun. Conf. (GLOBECOM), Madrid, Spain, Dec. 2021.
Wireless Communications via Intelligent Reflecting Surfaces
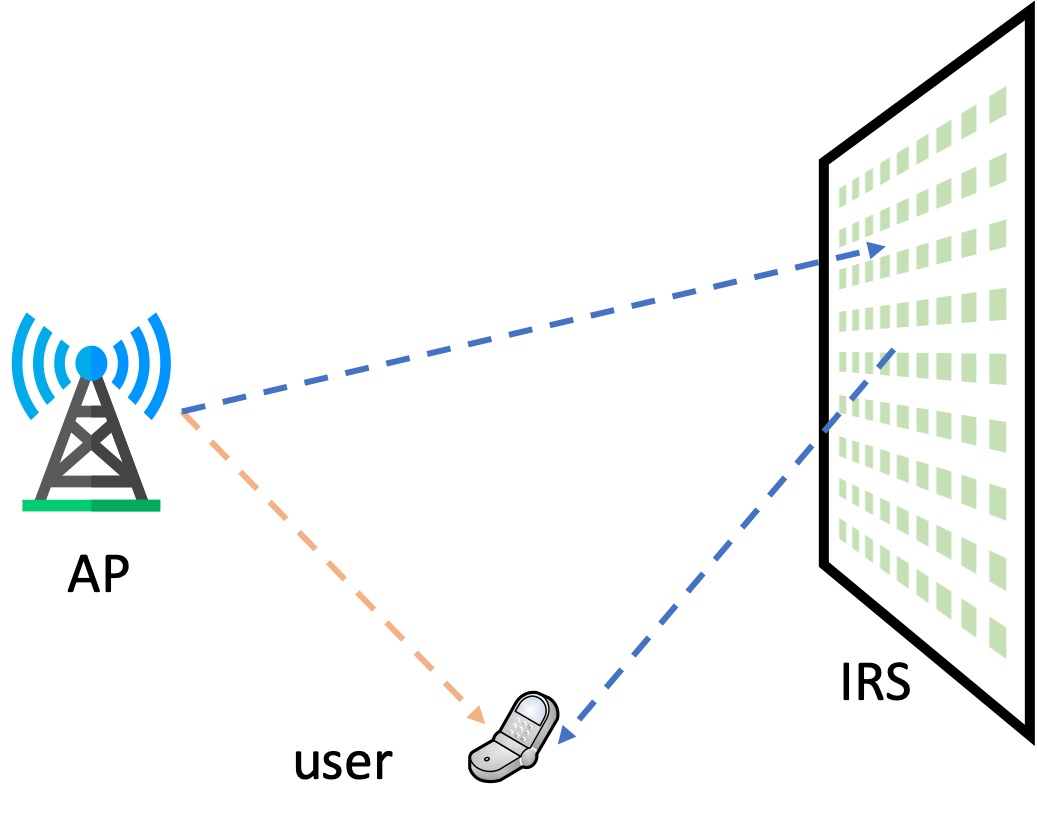
Recent advances in electromagnetic (EM) metasurfaces enable the manipulation of the impinging EM waves, which creates the possibility of controlling the wireless channel. Intelligent reflecting surfaces (IRSs), as a kind of passive metasurfaces, have been incorporated into wireless communications systems in recent years. In particular, IRSs are able to change the signal transmission direction with low-cost passive devices, e.g., printed dipoles and phase shifters, which is a revolutionary new characteristic that is not leveraged in conventional wireless communications systems. In this way, IRSs are able to create favorable wireless propagation environments without consuming power. Furthermore, IRSs can be readily coated on the facades of buildings, which reduces implementation cost and complexity. To fully exploit the potential of energy-efficient IRSs, they have to be properly designed and integrated with conventional communication techniques.
Q. Wu, T. Lin, X. Yu, Y. Zhu, and R. Schober, “Beamforming for PIN diode-based IRS-assisted systems under a phase shift-dependent power consumption model,” IEEE Trans. Commun., to appear. [Paper]
D. Xu, D. Shao, X. Yu, D. W. K. Ng, and R. Schober, “Multi-objective optimization for active IRS-assisted communication systems,” in Proc. IEEE Int. Mediterranean Conf. Commun. Netw. (MeditCom), Dubrovnik, Croatia, Sept. 2023, pp. 340-345.
X. Zhang, X. Yu, and S.H. Song, “Outage probability and finite-SNR DMT analysis for IRS-aided MIMO systems: How large IRSs need to be?” IEEE J. Sel. Topics Signal Process., Special Issue on Advanced Signal Processing for Reconfigurable Intelligent Surface-aided 6G Networks, May 2022, vol. 16, no. 5, pp. 1070-1085, Aug. 2022. [Paper]
T. Lin, X. Yu, Y. Zhu, and R. Schober, “Channel estimation for IRS-assisted millimeter-wave MIMO systems: Sparsity-inspired approaches,” IEEE Trans. Commun., vol. 70, no. 6, pp. 4078-4092, June 2022. [Paper]
X. Zhang, X. Yu, S.H. Song, and K. B. Letaief, “Large-scale IRS-aided MIMO over double-scattering channel: An asymptotic approach,” in Proc. IEEE Wireless Commun. Netw. Conf. (WCNC), Austin, TX, USA, Apr. 2022, pp. 2076-2081.
D. Xu, V. Jamali, X. Yu, D. W. K. Ng, and R. Schober, “Optimal resource allocation design for large IRS-assisted SWIPT systems: A scalable optimization framework,” IEEE Trans. Commun., vol. 70, no. 2, pp. 1423-1441, Feb. 2022. [Paper]
X. Yu, V. Jamali, D. Xu, D. W. K. Ng, and R. Schober, “Smart and reconfigurable wireless communications: From IRS modeling to algorithm design,” IEEE Wireless Commun., vol. 28, no. 6, pp. 118-125, Dec. 2021. [Paper]
D. Xu, X. Yu, D. W. K. Ng, and R. Schober, “Resource allocation for active IRS-assisted multiuser communication systems,” in Proc. Asilomar Conf. Signals, Systems, Computers, Pacific Grove, CA, USA, Nov. 2021, pp. 113-119. (Invited Paper) [Paper]
X. Yu, D. Xu, D. W. K. Ng, and R. Schober, “IRS-assisted green communication systems: Provable convergence and robust optimization,” IEEE Trans. Commun., vol. 69, no. 9, pp. 6313-6329, Sept. 2021. [Paper]
D. Xu, X. Yu, V. Jamali, D. W. K. Ng, and R. Schober, “Resource allocation for large IRS-assisted SWIPT systems with non-linear energy harvesting model,” in Proc. IEEE Wireless Commun. Netw. Conf. (WCNC), Nanjing, China, Mar. 2021, pp. 1-7.
D. Xu, X. Yu, Y. Sun, D. W. K. Ng, and R. Schober, “Resource allocation for IRS-assisted full-duplex cognitive radio systems,” IEEE Trans. Commun., vol. 68, no. 12, pp. 7376-7394, Dec. 2020. [Paper]
Y. Ma, Y. Shen, X. Yu, J. Zhang, S.H. Song, and K. B. Letaief, “A low-complexity algorithmic framework for large-scale IRS-assisted wireless systems,” in Proc. IEEE Global Commun. Conf. Wkshps. (GLOBECOM Wkshps), Taiwan, Dec. 2020, pp. 1-6.
X. Yu, D. Xu, D. W. K. Ng, and R. Schober, “Power-efficient resource allocation for multiuser MISO systems via intelligent reflecting surfaces,” in Proc. IEEE Global Commun. Conf. (GLOBECOM), Taiwan, Dec. 2020, pp. 1-6.
T. Lin, X. Yu, Y. Zhu, and R. Schober, “Channel estimation for intelligent reflecting surface-assisted millimeter wave MIMO systems,” in Proc. IEEE Global Commun. Conf. (GLOBECOM), Taiwan, Dec. 2020, pp. 1-6.
X. Yu, D. Xu, Y. Sun, D. W. K. Ng, and R. Schober, “Robust and secure wireless communications via intelligent reflecting surfaces,” IEEE J. Sel. Areas Commun., Special Issue on Wireless Networks Empowered by Reconfigurable Intelligent Surfaces, vol. 38, no. 11, pp. 2637-2652, Nov. 2020. (2023 IEEE Communications Society Leonard G. Abraham Prize) (ESI Highly Cited Paper) [Paper]
D. Xu, X. Yu, and R. Schober, “Resource allocation for intelligent reflecting surface-assisted cognitive radio networks,” in Proc. IEEE Int. Workshop Signal Process. Adv. Wireless Commun. (SPAWC), Atlanta, GA, USA, May 2020, pp. 1-5. (Invited Paper) [Paper]
X. Yu, D. Xu, and R. Schober, “Optimal beamforming for MISO communications via intelligent reflecting surfaces,” in Proc. IEEE Int. Workshop Signal Process. Adv. Wireless Commun. (SPAWC), Atlanta, GA, USA, May 2020, pp. 1-5. (Invited Paper) [Paper]
D. Xu, X. Yu, and R. Schober, “Resource allocation for secure IRS-assisted multiuser MISO systems,” in Proc. IEEE Global Commun. Conf. Wkshps. (GLOBECOM Wkshps), Waikoloa, HI, USA, Dec. 2019, pp. 1-6.
X. Yu, D. Xu, and R. Schober, “Enabling secure wireless communications via intelligent reflecting surfaces,” in Proc. IEEE Global Commun. Conf. (GLOBECOM), Waikoloa, HI, USA, Dec. 2019, pp. 1-6. (Best Paper Award) (IEEE ComSoc Best Readings) [Paper]
X. Yu, D. Xu, and R. Schober, “MISO wireless communication systems via intelligent reflecting surfaces,” in Proc. IEEE/CIC Int. Conf. Commun. China (ICCC), Changchun, China, Aug. 2019, pp. 735-740. (Invited Paper) [Paper]
Hybrid Precoding/Beamforming in Millimeter-Wave MIMO Systems
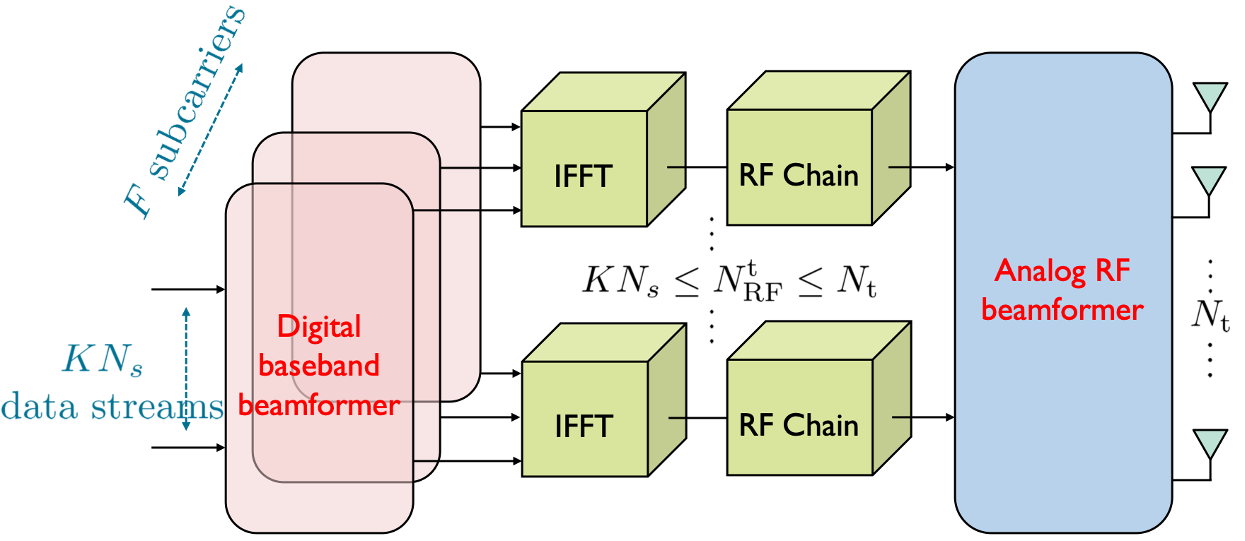
For traditional MIMO systems, precoding is typically accomplished at baseband through digital precoders, which can adjust both the magnitude and phase of signals. However, fully digital precoding demands radio frequency (RF) chains comparable in number to the antenna elements, which makes it infeasible at mm-wave bands. Given such unique constraints in mm-wave MIMO systems, a hybrid precoding architecture has recently received much consideration, which only requires a small number of RF chains interfacing between a low-dimensional digital precoder and a high-dimensional analog precoder.
C. Qi, Q. Liu, X. Yu, and G. Y. Li, “Hybrid precoding for mixture use of phase shifters and switches in mmWave massive MIMO,” IEEE Trans. Commun., vol. 70, no. 6, pp. 4121-4133, June 2022. [Paper]
Q. Liu, C. Qi, X. Yu, and G. Y. Li, “MmWave MIMO hybrid precoding design using phase shifters and switches,” in Proc. IEEE Global Commun. Conf. (GLOBECOM), Madrid, Spain, Dec. 2021, pp. 1-6.
J. Zhang, X. Yu, and K. B. Letaief, “Hybrid beamforming for 5G and beyond millimeter-wave systems: A holistic view,” IEEE Open J. Commun. Soc., vol. 1, pp. 77-91, Dec. 2019. (Invited Paper) [Paper]
X. Yu, J. Zhang, and K. B. Letaief, “Doubling phase shifters for efficient hybrid precoding in millimeter-wave multiuser OFDM systems,” J. Commun. Inf. Netw., vol. 4, no. 2, pp. 51-67, July 2019. [Paper]
X. Yu, J. Zhang, and K. B. Letaief, “A hardware-efficient analog network structure for hybrid precoding in millimeter wave systems,” IEEE J. Sel. Topics Signal Process., Special Issue on Hybrid Analog-Digital Signal Processing for Hardware-Efficient Large Scale Antenna Arrays, vol. 12, no. 2, pp. 282-297, May 2018. [Paper] [Codes]
X. Yu, J. Zhang, and K. B. Letaief, “Hybrid precoding in millimeter wave systems: How many phase shifters are needed?” in Proc. IEEE Global Commun. Conf. (GLOBECOM), Singapore, Dec. 2017, pp. 1-6. (Best Paper Award) [Paper]
X. Yu, J. Zhang, and K. B. Letaief, “Partially-connected hybrid precoding in mm-wave systems with dynamic phase shifter networks,” in Proc. IEEE Int. Wkshp. Signal Process. Adv. Wireless Commun. (SPAWC), Sapporo, Japan, July 2017, pp. 129-133.
X. Yu, J. Zhang, and K. B. Letaief, “Alternating minimization for hybrid precoding in multiuser OFDM mmWave systems,” in Proc. Asilomar Conf. Signals, Systems, Computers, Pacific Grove, CA, USA, Nov. 2016, pp. 1-5. (Invited Paper) [Paper]
X. Yu, J.-C. Shen, J. Zhang, and K. B. Letaief, “Alternating minimization algorithms for hybrid precoding in millimeter wave MIMO systems,” IEEE J. Sel. Topics Signal Process., Special Issue on Signal Processing for Millimeter Wave Wireless Communications, vol. 10, no. 3, pp. 485-500, Apr. 2016. (2018 IEEE Signal Processing Society Young Author Best Paper Award) (ESI Highly Cited Paper) (Top 25 downloaded article in 2019) [Paper] [Codes]
X. Yu, J.-C. Shen, J. Zhang, and K. B. Letaief, “Hybrid precoding design in millimeter wave MIMO systems: An alternating minimization approach,” in Proc. IEEE Global Commun. Conf. (GLOBECOM), San Diego, CA, USA, Dec. 2015, pp. 1-6.
Stochastic Geometry Analysis of Wireless Networks
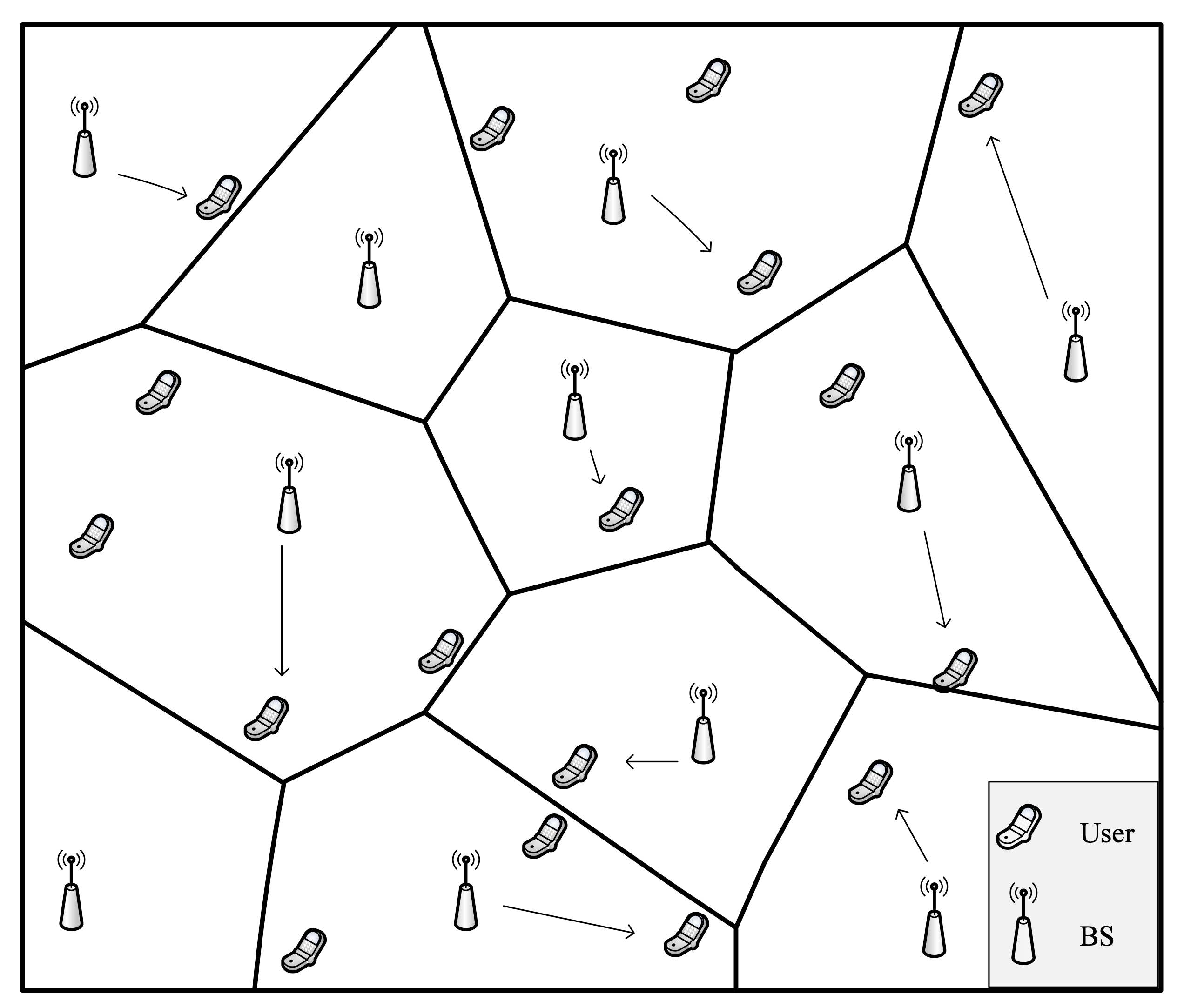
Besides increasing the carrier frequency, the other two main approaches to boosting the network capacity are to deploy multiple antennas for achieving high spectral efficiency and to deploy more access points per unit area in the network. It is crucial and intriguing to investigate and characterize the performance of multi-antenna wireless networks. As field trials are costly and system-level simulations are time-consuming, an effective alternative is to evaluate the network performance analytically with the assistance of a mathematical model. Stochastic geometry, especially point process theory, has recently been applied to wireless networks to model the spatial distribution of transceivers. Based on results derived with the help of stochastic geometry, abundant system insights can be obtained analytically, and provide critical guidance for network deployment, management, and optimization.
X. Yu, C. Li, J. Zhang, and K. B. Letaief, Stochastic Geometry Analysis of Multi-Antenna Wireless Networks, Springer, 2019. [Book Website]
X. Yu, J. Zhang, R. Schober, and K. B. Letaief, “A tractable framework for coverage analysis of cellular-connected UAV networks,” in Proc. IEEE Int. Conf. Commun. Wkshps. (ICC Wkshps), Shanghai, China, May 2019, pp. 1-6.
X. Yu, C. Li, J. Zhang, M. Haenggi, and K. B. Letaief, “A unified framework for the tractable analysis of multi-antenna wireless networks,” IEEE Trans. Wireless Commun., vol. 17, no. 12, pp. 7965-7980, Dec. 2018. [Paper]
X. Yu, J. Zhang, M. Haenggi, and K. B. Letaief, “Coverage analysis for millimeter wave networks: The impact of directional antenna arrays,” IEEE J. Sel. Areas Commun., Special Issue on Millimeter Wave Communications for Future Mobile Networks, vol. 35, no. 7, pp. 1498-1512, July 2017. (ESI Highly Cited Paper) [Paper]
X. Yu, C. Li, J. Zhang, and K. B. Letaief, “A tractable framework for performance analysis of dense multi-antenna networks,” in Proc. IEEE Int. Conf. Commun. (ICC), Paris, France, May 2017, pp. 1-6.
X. Yu, J. Zhang, and K. B. Letaief, “Coverage analysis for dense millimeter wave cellular networks: The impact of array size,” in Proc. IEEE Wireless Commun. Netw. Conf. (WCNC), Doha, Qatar, Apr. 2016, pp. 1-6.
Others
H. He, X. Yu, J. Zhang, S.H. Song, and K. B. Letaief, “Cell-free ultra-massive MIMO detection: A distributed expectation propagation approach,” submitted to IEEE Trans. Mobile Comput., Dec. 2024. [Paper]
X. Zhang, D. Xu, X. Yu, S.H. Song, and M. Debbah, “Finite blocklength analysis for optical fiber MIMO channels,” accepted to IEEE Global Commun. Conf. (GLOBECOM), Cape Town, South Africa, Dec. 2024, pp. 843-848.
Z. Wei, X. Yu, D. W. K. Ng, and R. Schober, “Resource allocation for simultaneous wireless information and power transfer systems: A tutorial overview,” Proc. IEEE, vol. 110, no. 1, pp. 127-149, Jan. 2022. (Invited Paper) [Paper]
H. He, X. Yu, J. Zhang, S.H. Song, and K. B. Letaief, “Cell-free massive MIMO for 6G wireless communication networks,” J. Commun. Inf. Netw., vol. 6, no. 4, pp. 321-335, Dec. 2021. (Invited Paper) [Paper]
H. He, H. Wang, X. Yu, J. Zhang, S.H. Song, and K. B. Letaief, “Distributed expectation propagation detection for cell-free massive MIMO,” in Proc. IEEE Global Commun. Conf. (GLOBECOM), Madrid, Spain, Dec. 2021, pp. 1-6.